top of page


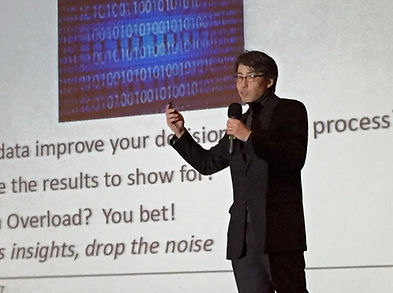
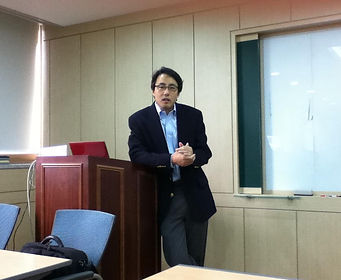
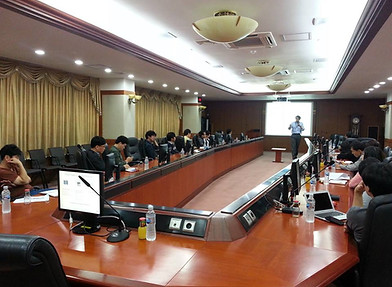
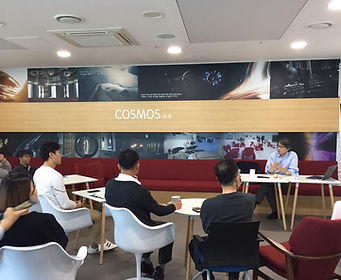
Articles and Presentations
English
Adweek (Monthly Series 10/2020-9/2022):
1: Why Data Scientists Must Act as Mediators and Translators Between IT and Marketing
2: How to Eliminate Emotional Factors From Predictive Analytics
3: The Right Answers Come Only From Asking the Right Questions
4: Why Data Presentation Needs to Be Digestible and Insightful
5: Marketers Should Use Modeling to Compress Large Data into Small Answers
6: Not All Data Types Are Equally Useful
7: Transaction Data Is the Most Powerful Predictor of Future Behavior
8: 14 Ways Brands Can Put One-to-One Marketing Back to Work
9: Personalization Is Not About Product, Channel or Brand
10: The First Rule of Personalization: How to Not Annoy the Customer
11: Personalization Is a Team Effort, and Technology Is Only One Player
12: Personalization Is a Long-term Commitment
13: Don't be Creepy: Personalization is about Gentle Nudging
14: Marketers Need to Understand the Difference Between Segments and Personas
15: Building a Persona Menu for Effective Messaging
16: Modeling Turns the Risky Unknown Into the Hopeful Potential
17: Connecting Online and Offline Customer Profiles to the Real World
18: Going Beyond Modeling to Find New Customer Acquisition Targets
19: From Reactive to Holistic: Exploring the Various Levels of Personalization
20: Not All Customer Data Is Equally Valuable
21: Abusers of Consumer Data Will Be Punished
Target Marketing Magazine (Monthly Series 11/2013-9/2020):
"Big Data, Small Data, Clean Data, Messy Data"
88 articles have been migrated to Adweek after their merger in 2020.
Click here for the full list.
Contact us to pick relevant articles for your specific needs.
2024 Data Strategy Presentation:
Monetizing Data Assets via Data Refinement and Analytics (PDF)
ANA Data & Analytics Practice Webinar 8/2023:
4 Pillars of Holistic Personalization in the Age of AI and ChatGPT (YouTube Link)
4 Pillars of Holistic Personalization in the Age of AI and ChatGPT (m4v Video)
4 Pillars of Holistic Personalization in the Age of AI and ChatGPT (PDF)
ANA Data & Analytics Practice Webinar 9/2022:
Customer Centric Data and Analytics Readiness (YouTube Link)
Customer Centric Data and Analytics Readiness (m4v Video)
Customer Centric Data and Analytics Readiness (PDF)
ANA Data & Analytics Practice Webinar 1/2022:
Road to Holistic Personalization (YouTube Link)
Road to Holistic Personalization (m4v Video)
Road to Holistic Personalization (PDF)
WVU Marketing Communications Today Podcast 1/2022:
It's Time to Humanize Data (Audio & Script)
Cipher Creative Group 11/2020:
The Four Pillars of Effective Personalization
- and When Analytics and Creativity Should Meet (YouTube Link)
Interview with Career in Analytics 6/2016:
Advice for Aspiring Data Scientists
insideBIGDATA Article 6/2015:
Data Refinement: The Dirty Side of Data Science
Print+Promo Interview 4/2015:
5 Ways to Tidy Up Your Data Strategy
TV Interview at the QIBRAS Conference in Sao Paulo 9/2014:
Success Factors of Data Strategy (YouTube Link)
Korean
중앙일보 전문가 칼럼 (2019년 8월 - 2020년 12월)
"유혁의 데이터 이야기"
1회: 빅 데이터로 재미 좀 보셨습니까?
2회: 사장님, 데이터 가지고 그러시면 안 됩니다
3회: 데이터를 잘 다루는 사람이란?
4회: 기계에게 목적을 부여하는 인간
5회: 훌륭한 데이터 사이언티스트는 만들어진다
6회: 데이터 사용하려는 목적부터 분명히 하라
7회: 데이터는 도구이지 공포의 대상이 아니다
8회: 데이터는 제대로 분석해야 맛이 난다
9회: 데이터 분석 결과는 단순한 의견이 아니다
10회: 데이터 가지고 거짓말하면 안됩니다
11회: 데이터 기반의 의사결정을 잘하는 방법
12회: 알고리즘이 지배하는 세상
13회: 할 수 있는 일이라고 다 해선 안 된다
14회: 빅데이터에는 상명하복이 통하지 않는다
15회: 데이터 기반 마케팅의 주체는 소비자다
16회: 데이터 분석가는 통역가이자 중재자 돼야
17회: 정보도 편식하면 안됩니다
18회: 데이터는 감정 빼고 봐야한다
19회: 잘못된 질문엔 답이 없다
유혁의 "데이터를 잘 써먹을 수 있는 구체적인 방법들" 단행본과 더불어 발간된 요약본
한국정보화진흥원(NIA) 발행 빅데이터 실무서 "데이터를 잘 써먹을 수 있는 구체적인 방법들" (단행본)
한국정보화진흥원(NIA) 발행 빅데이터 실무서 "빅데이터 - 질문을 명확히 하라" (요약본)
IT Daily의 소개기사 (2017년 2월 22일)
유혁의 IT Daily와 컴퓨터 월드 월간 연재 칼럼 (2014년 2월 - 2015년 8월)
"데이터를 잘 써먹을 수 있는 구체적인 방법들"
1회: 연재를 시작하면서 - 빅 데이터에 관한 생각들
3회: 빅 데이터의 핵심은 분석이며 분석의 중심은 모델링
8회: 자유형(Freeform) 데이터는 결코 공짜가 아니다
17회: 예측적 분석을 통한 소비자와의 Relevancy 유지
18회: 연재를 마치며 - Data Movement의 미래는?
IT Daily/컴퓨터 월드 주최 지상 좌담회 2015년 1월
"빅 데이터와 데이터 사이언티스트에 대한 15인 15색의 목소리"
IT Daily/컴퓨터 월드 주최 좌담회 2014년 11월
"정보의 흐름을 아는 사람이 가치를 창출한다" (지상중계)
IT Daily/컴퓨터 월드 주최 빅 데이터 컨퍼런스 기조연설 2014년 11월
"빅 데이터 성공의 조건은 무엇인가" (관련기사)
IT Daily/컴퓨터 월드 주최 빅 데이터 컨퍼런스 기조연설 2013년 11월
"빅 데이터에 관한 생각들" (비디오)
IT Daily/컴퓨터 월드 빅 데이터에 관한 대담 2013년 10월:
"빅 데이터는 단지 지나가는 유행이 아니라 접근법 간의 벽을 허무는 과정"
Stephen H. Yu Photo: IT DAILY/COMPUTER WORLD

bottom of page